Deriving at Software Decisions with AI (Transmission #279)
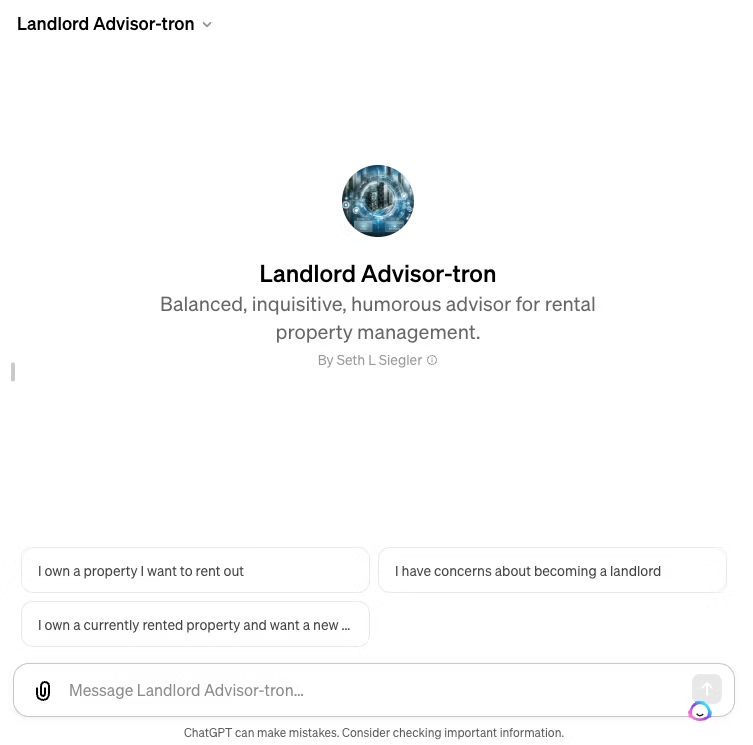
As always, links surrounded by the ❇️ emoji indicate exclusive GEM Diamond content. If you would like to have access to all links, please consider GEM Diamond membership.
Practicing agents, big and small landlords, and general contractors all have to evaluate the software that will serve as the spine of their business. Oh yeah, and consumers, too.
There’s too much noise, and it takes too much time to sift through it all. How do you decide which software to evaluate? Usually, it’s asking a few peers what they use. Those peers inherently have limited experiences to draw upon. Yes, a few might try and test multiple products and services before eventually settling on a winner. But, many just follow the herd as a lemming. If their friends use bad products, they will use those same bad products.
And the cycle continues.
What if there were a better way to evaluate critical software? One that alleviates the core “noise” problem? And actually helps the industry adopt better solutions?
CONVERSATIONAL, BUT KNOWLEDGEABLE
Enter custom GPTs. Announced late last year by OpenAI, these are “a variation of the GPT (Generative Pre-trained Transformer) model, like ChatGPT, that has been customized or fine-tuned for specific applications or use cases.”
How could it be used to help a customer segment evaluate potential solutions?
Give the custom GPT a list of all the companies in a sector using the "Actions" feature of GPT's, which would allow it to call an API. The GPT would then index those specific URLs and limit its answers to that baseline training material—when paired with a scraping API service (such as ScraperAPI), the custom GPT could maintain real-time knowledge on a vast array of updates and services from the sector’s vendors.
Or, you could even build a basic app that is it's own scraper—that app can then index the vendor websites daily, check for updates or changes, store that data in it's own normalized database, and then expose an API for your app to your custom GPT via the "actions" option too.
Alternatively, a quick and dependable path would mean saving the key pages of each site as PDF and then uploading them as "knowledge" in your GPT. This would require some upkeep to remain current, but would maximize accuracy.
A customer would then provide information about their use case into the conversation chat experience: where they operate, what problems they are trying to solve, etc.
Pretty simple, at least to start. The GPT owner sourcing and ingesting reviews and documented learnings from customers asking targeted questions into the knowledge base would improve the quality, as well as adding independent articles to the knowledge base the GPT is learning from that describe companies, use cases, and customer specific examples would hone the decision engine’s abilities further. The problems arising from the inflated capabilities listed on product splash pages can be left for another day.
Curious? Additional examples.
Proptech's Leader has Been Crashing Our Couch the Whole Time // Stock in Down Payments // Banking Real Estate in the Clouds